Testing in a Pandemic
25 May 2020|
- Research
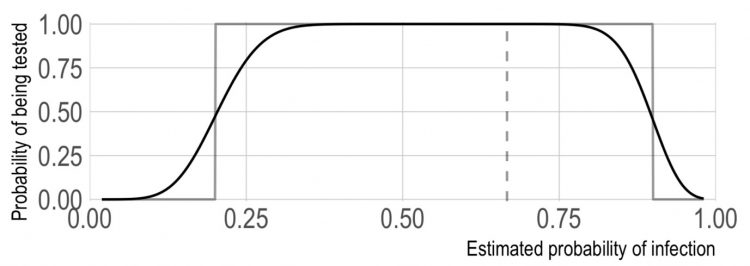
What do we want, and when do we want it? What is the value of information? What should we do to learn about the world? How does that depend on our normative assumptions? These are some of the fundamental questions of scientific method, but also of artificial intelligence. These questions motivate many of my research projects, on topics such as experimental design, or the reform of the scientific publication system.
These questions can become a matter of life and death in a pandemic such as the current one, when capacity for infectious disease testing is limited. My co-author Alex Teytelboym and I have therefore just written a piece on the optimal use of limited testing resources, which can be found here, and which I will briefly discuss in this post.
Testing is a critical part of a response to an epidemic. At an individual level, testing allows authorities to identify and quarantine sick people, thereby stopping the spread of the disease. At a country level, testing helps authorities keep track of the disease spread, make decisions about social distancing rules, and plan for provision of supplies. However, during a sudden epidemic, such as COVID-19, testing resources can be limited. Evidence from the COVID-19 pandemic suggests that countries had very different testing capacities. Even if testing kits themselves are cheap, large-scale laboratory testing capacity might be infeasible or it might be difficult to quickly reach all those who need testing. In our paper, we offer a simple framework that formalises the key trade-offs that policymakers might face under limited testing capacity and propose an adaptive policy that can help them allocate their testing capacity as effectively as possible.
We work under the assumption that the policymaker’s objective is to minimize the total cost of disease spread. These costs are of many kinds: cost of human lives, cost of lost labour income, cost of testing kits, and reputation cost of unnecessary quarantine. In our model, if the policymaker decides to test an individual, they incur a testing cost, but they will subsequently take the right quarantining decision. If the policymaker decides not to test the individual, they can make one of two costly errors:
- False Quarantine: Quarantining an individual who is not infected.
- False Release: Not quarantining an individual who is infected.
During the COVID-19 pandemic, countries appear to have used their testing capacity in different ways. For example, there is a substantial variation in the number of confirmed cases per test even after controlling for prevalence and testing capacity. The question we answer in this paper is: What is the testing policy that minimises the overall costs?
The following example elucidates the key tradeoffs. Suppose that the policymaker only has 10,000 testing kits, but there are 20,000 individuals who have arrived at the hospital. Whom should the policymaker test? Consider two policies that have been used repeatedly in the current pandemic.
Priority Testing: Rank all individuals according to how likely they are to have the disease. Then test 10,000 people who are most likely to have the disease.
Several countries, such as the United States and United Kingdom, implicitly used the Priority Testing policy during the initial stages of COVID-19 pandemic by restricting testing to patients with strong symptoms, to those who travelled to infected area or to those who have been in contact with infected people.
Priority Testing might be the optimal policy only if the cost of falsely quarantining individuals who are not infected is extremely high. But by testing individuals that are likely to have the disease, the policymaker could potentially be “wasting” tests: if the cost of a false quarantine errors is not too high, the people with the highest estimated likelihood of the disease could be quarantined without testing. During the COVID-19 pandemic, many countries eventually followed this logic and advised that anyone who has symptoms or who lives with someone who has symptoms of COVID-19 must self-isolate without testing for an extended period.
Random Testing: Test 10,000 individuals at random.
During the COVID-19 pandemic, a few countries and cities used random testing and there have been several calls to expand Random Testing. Random Testing is a sensible policy if tests are very cheap. The policymaker can learn the prevalence of the disease (thereby being able to make better decisions about testing of individuals in the future), but most people tested will not be infected. Therefore, many tests will, once again, be “wasted”.
In our paper, we first point out that to make optimal decisions about testing the policymaker needs to trade off the costs of false quarantine and false release relative to the cost of testing. Under fairly mild conditions, the optimal myopic testing policy is to test individuals with an intermediate likelihood of the disease. Priority Testing is therefore not myopically optimal in general because the policymaker would prefer to quarantine individuals with a high likelihood of infection without testing them and would not test or quarantine individuals who are very unlikely to be infected.
We then look at how the policymaker’s problem changes when she cares about the future. In this case, we show that the policymaker will not initially want to follow the myopic policy. Rather the policymaker would want to “explore” by initially spreading out some of her testing capacity and sacrificing some immediate benefit. The reason is that such exploratory testing gives the policymaker valuable information about the prevalence of the disease which she can use to make better decisions about the testing of future individuals. A simple dynamic testing policy, derived from an old recommendation by Thompson (1933), tells the policymaker how much exploration is (nearly) optimal. The Thompson policy starts by initial exploratory testing. If the prevalence rate is stable, the payoff to exploration disappears as the number of tested individuals grows because disease prevalence becomes precisely estimated. Over time, the Thompson policy converges to the optimal myopic testing policy.
Category: Research